Machine learning has quickly become the focal point of the computing industry. Whether this branch of Artificial Intelligence is implementing deep learning, reinforcement learning, supervised learning, or another approach—the applications seem limitless.
With this surge in popularity has come a surge in demand for skilled machine learning professionals. Whether you are studying machine learning for the first time, honing your skills before the job hunt, or a professional continuing their study of AI—books can help.
Introduction
The need for machine learning and artificial intelligence references is at a peak in demand. This demand reflects both increased interests among academia and commercial demands for hiring. There has been a corresponding increase in academic programs in fields related to computer science, programming, and artificial intelligence (3).
The need for quality and comprehensive reference materials to assist in learning, training, and expanding the skillsets of ML and AI programmers is essential. The aim of this analysis was to compile a list of ML and AI book titles that are recommended by URLs appearing in Google searches for keyword phrases related to Machine learning and Artificial intelligence books.
This article details the methods and results of this analysis. For a listing of the book titles produced from this survey please see the review article here: Best Machine Learning & Artificial Intelligence Books.
Methods
A list of URLs containing lists of book recommendations was analyzed to gather unique titles of books, as determined by ISBN-13 (1) identifiers. These URL sources for these recommendations were collected through a series of Google searches using keyword phrases related to an initial seed keyword phrase.
Related keywords were prioritized in descending order of estimated monthly search volume in the US-Google database. Information related to related keywords and search volumes was provided by the SEMRush service (2). The analysis of URLs with book recommendation lists is detailed below as a series of six unique phases of analysis and quantification.
Phases:
- Keyword Discovery
- Keyword prioritization
- Relevant URL identification
- Book Title Discovery
- Book Title Filtering/Exclusions
- Book Title Frequency Sorting
Keyword Discovery
The SEMRush marketing platform was used to identify target keywords related to recommended book titles on machine learning and artificial intelligence. An initial keyword search using the phrase “best machine learning books” was searched in the US database of the Google search engine.
The URLs from the top 5 organic results of this search were obtained as seed URLs to gather related keyword terms to broaden search parameters for later searches. The SEMRush marketing platform was then used to gather search data related to total organic keywords and estimated monthly search traffic for these URLs.
Filtering was applied to the organic position reporting data for each URL to ensure maximal relevancy. A positional filter (P) was used to eliminate keywords where the URL did not appear in an organic result at or below the 20th position. A volume-based sorting filter (V) was also applied to sort results based on the largest estimated monthly search interest. The results obtained from the initial search are reported in Table 1 and Table 2.
Keyword Prioritization
The organic keyword results for each URL were filtered for those where the pages appeared within the top 20 results. This filter was applied to remove unrelated keyword phrases from reporting data.
The organic keyword results were then sorted in descending order of volume (V) to identify the phrases that represented the largest numbers of monthly searches—a proxy for interest. Results of this initial search can be found in Table 2.
Relevant URL Identification
The top 10 keywords resulting from the keyword prioritization phase were used as seed keywords to conduct a series of Google searches. For each keyword among these 10, a unique Google search in the US database was performed to produce a unique search engine results page (SERP) from which the resulting top 5 organic page URLs were recorded. This process produced a set of 33 unique URLs.
Book Title Discovery
The 33 unique URLs were then manually inspected to assess relevancy for containing book titles. Of the 33 URLs discovered only 16 were deemed relevant to book title discovery. URLs were disqualified via a manual inspection process on the grounds of either being relevant to only a single book title or not mentioning book titles. This represented a ~49% relevancy rate among URL inclusions.
Relevant URLs were then manually inspected for book titles and any titles found included were added to a global list of unique titles, up to a maximum of 10 titles per URL source. This upper limit was put into place after observing several URLs to contain excessive numbers of relative titles. Where titles were found to be uniquely identified only by edition, all but the most recent edition were excluded. Another series of filtering for uniqueness was done by ISBN comparison when that data was available. Amazon.com was used as the source for ISBN listings.
Exclusion Criteria
Given the statistical nature of this study, it was deemed appropriate to include as many recommendations from as many sources as practical. As such, many titles that were regarded as possibly irrelevant were included for consideration. The frequency of occurrence among several sources was maintained as an effective filter for excluding such titles.
Titles without an ISBN number were excluded. In such cases, these entries were considered non-entries and an additional entry was considered from the list where available. There was only one instance in which this exclusionary filter was applied to a book titled Monroe Doctrine II. This book was categorized as fiction.
In the case of Amazon best-seller category lists, some titles appeared multiple times within the top 10 results as multiple formats. For example, the title “Life 3.0: Being Human in the Age of Artificial Intelligence” appeared both in the Kindle edition and Paperback edition. In this case, as in the case of similar duplicates, the physical version was selected and an additional entry was taken from the list.
An additional series of exclusions were made after the titles were collected and frequency analysis was performed. This exclusion removed book titles appearing on fewer than 3 URLs. This filtering was done after noting that, in addition to 61 titles appearing on single URLs only, there were ample titles appearing on 3 or more sources to compile an adequate list.
Title Frequency Sorting
The results from the title gathering process were then analyzed for frequency of mention among all considered recommending URLs. The list of book titles and corresponding frequency counts were then sorted in descending order to provide a list of the most frequent titles among all URLs recommending books. This ordered data then served as our basis for the list of best books on machine learning and artificial intelligence.
Results
The total organic keywords after having the positional filter applied (P) and the total estimated monthly traffic of the top 5 organic URLs returned for the key term phrase “best machine learning books” are reported in Table 1.
These results reflect known traffic distribution patterns of Google search results such that higher results receive roughly 35% of total clicks resulting in greater estimated monthly traffic counts.
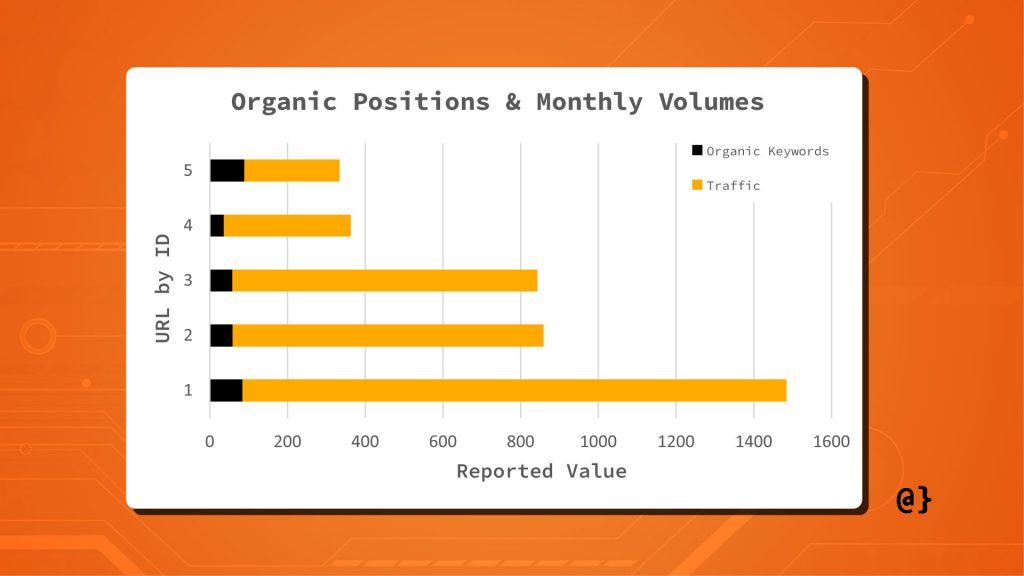
After the collection of organic keyword data for each of these initial 5 URLs, a set of unique keywords found among all URLs was collected. This set was then sorted based on total monthly volume to, again, serve as a proxy representing cumulative interest. There were no filtering criteria applied for inclusion other than the appearance on any of the 5 URLs.
This collection was then sorted in by monthly search volume in descending order. The keywords reflecting the 10 highest monthly search volumes were then selected as the keyword phrases URL discovery in the book title gathering phase. These results are reported in Table 2 below. A JSON-format file containing the collection of all keywords, their relative counts among the considered URLs, and the monthly volumes are available here for download.

Of the unique URLs found within the SERP for these terms, a total of 148 book titles were discovered resulting in 89 unique titles for consideration. Of these 89 titles, only 28 appeared on more than one URL. This number was deemed impractical for representing a general consensus among considered URLs such that only book titles appearing on 3 or more distinct URL lists were included. A breakdown of these results is illustrated in Table 3.
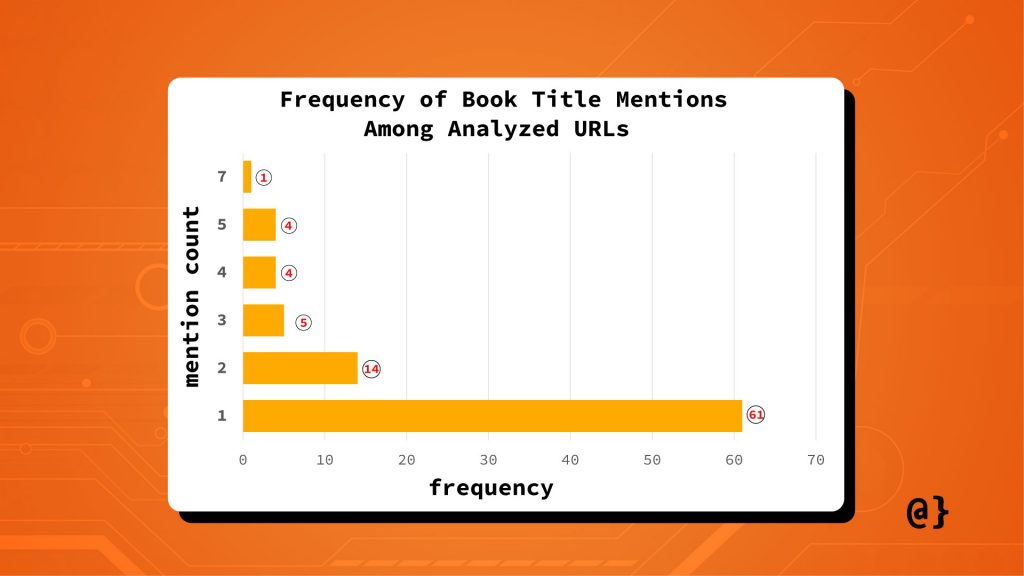
There were a total of 14 unique titles After the initial frequency analysis and resulting exclusionary filtering. This represents our “top n list” resulting from functional consensus inference among the URLs considered. A breakdown of the titles and related frequency recordings is shown in table 4.

Discussion
Total monthly search volume was used for keywords as a functional proxy to represent a total interest in a particular keyword phrase. This was an arbitrary assumption without basis in any objective reporting.
Worth noting is the several possible improvements in the process for similar research in the future. The utility of applying a similarity filter on keywords such that “machine learning books” and “best machine learning books” could be consolidated into a single result. Google was observed to provide results filtered similar to this idea but no analysis was performed to assess to what degree.
A simple cosine similarity function could produce a result such that, for each keyword, if any other keyword were found to be within a range of similarity one or the other would be chosen, based on criteria. One example criterion would be the more specific (longer) of the two. Recommending sorting approaches would be in descending order of length prior to comparison such that a graduated progression.
This approach could then apply similarity thresholds i.e. a two-word keyword phrase like “machine learning” such that “machine learning book” would be replaced the active search term with the more specific key terms. This could then be compared for similarity against more specific terms such as “best machine learning textbook.” Without a graduated approach based on length, similarity scoring for shorter words and longer words would not likely be identified.
Also worth noting is that that no filtering criteria were applied during the collection of unique keyword phrases among the initial 5 URLs. Relevancy would be improved by implementing a filter requiring any keyword considered for final analysis to have appeared on at least n-many URLs for inclusions. This would serve to greater reflect consensus among different URLs.
The nature of titles seemed to vary among sources, though remained consistent for each. For example, certain URLs recommended machine learning and artificial intelligence books that were predominantly narrative while others recommended titles that were predominantly technical or academic in nature.
Future studies could benefit from using more empirical inclusion criteria for limiting the number of titles included from a specific URL. For example, a list of total titles could be collected making note of the count on a per-URL basis. A basic statistical distribution could then be calculated and used to filter based on standard error distance relative to the mean title counts of all URLs.
Conclusion
Compiling a list of the most recommended machine learning and artificial intelligence books serves as a functional proxy for a crowd-sourced “best of” list. The sheer number of unique book titles found in the discovery process reflects how diverse the field of Machine Learning and Artificial Intelligence is.
The titles in this survey reflect a diverse perspective on the subject matter as well—inclusive of scholastic texts, practical guides, and narrative works touching on both relevant philosophies and ethical considerations of the fields.
Possible future analysis would benefit from polling ML and AI-centric communities, academic departments, and large datasets such as the Reddit or Quora comment corpora. Such additions could stand as separate collections or be integrated with current titles via weighted means to reflect novel presentation.
References
- Information and documentation — International Standard Book Number (ISBN). ISO 2108:2017. https://www.iso.org/standard/65483.html
- Semrush.com. 2021. Organic Research: Keyword Rank Checker. Available at: https://www.semrush.com/kb/20-organic-research [Accessed 6 July, 2021]
- Daniel Zhang, et. al, “The AI Index 2021 Annual Report,” AI Index Steering
Committee, Human-Centered AI Institute, Stanford University, Stanford, CA, March 2021.